Object Detection using Feature Mining in a Distributed Machine Learning Framework
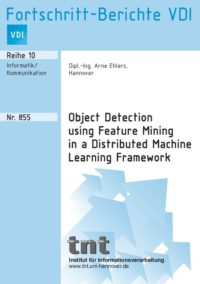
Erscheinungsdatum: 07.08.2017
Reihe: 10
Band Nummer: 855
Autor: Dipl.-Ing. Arne Ehlers
Ort: Hannover
ISBN: 978-3-18-385510-0
ISSN: 0178-9627
Erscheinungsjahr: 2017
Anzahl Seiten: 162
Anzahl Abbildungen: 72
Anzahl Tabellen: 10
Produktart: Buch (paperback, DINA5)
Produktbeschreibung
This dissertation addresses the problem of visual object detection based on machine-learned classifiers. A distributed machine learning framework is developed to learn detectors for several object classes creating cascaded ensemble classifiers by the Adaptive Boosting algorithm. Methods are proposed that enhance several components of an object detection framework: At first, the thesis deals with augmenting the training data in order to improve the performance of object detectors learned from sparse training sets. Secondly, feature mining strategies are introduced to create feature sets that are customized to the object class to be detected. Furthermore, a novel class of fractal features is proposed that allows to represent a wide variety of shapes. Thirdly, a method is introduced that models and combines internal confidences and uncertainties of the cascaded detector using Dempster’s theory of evidence in order to increase the quality of the post-processing.
Keywords: Object Detection, Feature Mining, Fractal Features, Data Augmentation, Machine Learning, Adaptive Boosting, Distributed Computing, Object Detection, Feature Mining, Fractal Features, Data Augmentation, Machine Learning, Adaptive Boosting, Distributed Computing
* Der VDI-Mitgliedsrabatt gilt nur für Privatpersonen