Plant Classification and Position Estimation for Autonomous Field Robots
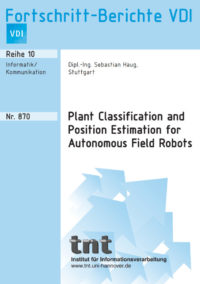
Erscheinungsdatum: 08.12.2020
Reihe: 10
Band Nummer: 870
Autor: Dipl.-Ing. Sebastian Haug
Ort: Stuttgart
ISBN: 978-3-18-387010-3
ISSN: 0178-9627
Erscheinungsjahr: 2020
Anzahl Seiten: 164
Anzahl Abbildungen: 85
Anzahl Tabellen: 15
Produktart: Buch (paperback, DINA5)
Produktbeschreibung
This work presents new approaches to plant classifcation and plant position estimation to enable feld robot based precision agriculture. The developed methods are designed for challenging real world feld situations with small crop plants, presence of close-to-crop weed and overlap of plants. The plant classifcation system is able to distinguish two or more plant classes in feld images without the need for error-prone plant or leaf segmentation. The plant
position estimation pipeline solves the generic problem of determining the position of both crop and weed plants only from image data. The combination of both methods allows feld robots to autonomously determine the type and position of plants in the feld to realize precision agriculture tasks such as single plant weed control. Experiments with a feld robot prove the applicability of the presented methods for challenging feld scenarios encountered for example in organic vegetable farming.
Contents
Symbols and Abbreviations . . . . . . . . . . . . . . . . . . . . . . . . . . . . . . . . . . . VII
1 Introduction 1
1.1 Scope . . . . . . . . . . . . . . . . . . . . . . . . . . . . . . . . . . . . . . . . . . . . . . . . . . . . 2
1.2 Related Work . . . . . . . . . . . . . . . . . . . . . . . . . . . . . . . . . . . . . . . . . . . . . . 3
1.3 Contributions of the Thesis . . . . . . . . . . . . . . . . . . . . . . . . . . . . . . . . . . . . 4
1.4 Structure of the Thesis . . . . . . . . . . . . . . . . . . . . . . . . . . . . . . . . . . . . . . . 6
2 Background: Computer Vision and Machine Learning 9
2.1 Computer Vision . . . . . . . . . . . . . . . . . . . . . . . . . . . . . . . . . . . . . . . . . . . 9
2.2 Machine Learning . . . . . . . . . . . . . . . . . . . . . . . . . . . . . . . . . . . . . . . . . . . 13
2.3 Summary . . . . . . . . . . . . . . . . . . . . . . . . . . . . . . . . . . . . . . . . . . . . . . . . . 23
3 Multispectral Image Acquisition and Vegetation Segmentation 24
3.1 Multispectral Field Image Acquisition . . . . . . . . . . . . . . . . . . . . . . . . . . . . 24
3.2 Vegetation Segmentation . . . . . . . . . . . . . . . . . . . . . . . . . . . . . . . . . . . . . 30
3.3 Summary . . . . . . . . . . . . . . . . . . . . . . . . . . . . . . . . . . . . . . . . . . . . . . . . . 38
4 Plant Classification 40
4.1 Related Work . . . . . . . . . . . . . . . . . . . . . . . . . . . . . . . . . . . . . . . . . . . . . . 41
4.2 Novel Plant Classification Pipeline . . . . . . . . . . . . . . . . . . . . . . . . . . . . . . . 48
4.3 Offline Pipeline Training Steps . . . . . . . . . . . . . . . . . . . . . . . . . . . . . . . . . . 60
4.4 Evaluation Criteria . . . . . . . . . . . . . . . . . . . . . . . . . . . . . . . . . . . . . . . . . . 63
4.5 Parameter Selection . . . . . . . . . . . . . . . . . . . . . . . . . . . . . . . . . . . . . . . . . 63
4.6 Summary . . . . . . . . . . . . . . . . . . . . . . . . . . . . . . . . . . . . . . . . . . . . . . . . . 69
5 Plant Position Estimation 70
5.1 Related Work . . . . . . . . . . . . . . . . . . . . . . . . . . . . . . . . . . . . . . . . . . . . . . 71
5.2 Novel Plant Position Estimation Pipeline . . . . . . . . . . . . . . . . . . . . . . . . . . 76
5.3 Training Phase . . . . . . . . . . . . . . . . . . . . . . . . . . . . . . . . . . . . . . . . . . . . . 82
5.4 Evaluation Criteria . . . . . . . . . . . . . . . . . . . . . . . . . . . . . . . . . . . . . . . . . . 85
5.5 Parameter Selection . . . . . . . . . . . . . . . . . . . . . . . . . . . . . . . . . . . . . . . . . 87
5.6 Summary . . . . . . . . . . . . . . . . . . . . . . . . . . . . . . . . . . . . . . . . . . . . . . . . . 95
6 Experimental Results and Discussion 96
6.1 Data Acquisition Robot and Dataset Properties . . . . . . . . . . . . . . . . . . . . . 96
6.2 Evaluation and Discussion of the Plant Classification Method . . . . . . . . . . . 105
6.3 Evaluation and Discussion of the Plant Position Estimation Method . . . . . . 117
6.4 Combined System for Weed Control . . . . . . . . . . . . . . . . . . . . . . . . . . . . . 125
6.5 Summary . . . . . . . . . . . . . . . . . . . . . . . . . . . . . . . . . . . . . . . . . . . . . . 128
7 Conclusion. . . . . . . . . . . . . . . . . . . . . . . . . . . . . . . . . . . . . . . . . . . . . . 129
A Additional Results . . . . . . . . . . . . . . . . . . . . . . . . . . . . . . . . . . . . . . . . . . . . . . 133
A.1 Results for the Crop Weed Field Image Dataset . . . . . . . . . . . . . . . . . . . . . . 133
A.2 Plant Classification Parameter Selection for Dataset B . . . . . . . . . . . . . . . . 134
A.3 Plant Position Estimation Parameter Selection for Dataset B . . . . . . . . . . . . 136
Bibliography 139
Keywords: Bildverarbeitung, Maschinelles Lernen, Landwirtschaft, Robotik, Pflanzenklassifikation, Pflanzenpositionsschätzung, Unkrautregulierung, Computer Vision, Machine Learning, Agriculture, Robotics, Plant Classifcation, Plant Position Estimation, Weed Control
* Der VDI-Mitgliedsrabatt gilt nur für Privatpersonen